What Does Relationship Between Variables Mean
The relationship between variables refers to how the values of two or more variables correlate or interact with each other. Understanding the relationship between variables is crucial in various fields, including statistics, research, and data analysis.
It helps to determine if and how changes in one variable correspond to changes in another variable. This knowledge aids in predicting outcomes, making informed decisions, and identifying patterns or trends. By examining the relationship between variables, researchers can draw conclusions about cause and effect, assess the strength of the association, and explore potential dependencies.
Whether studying the impact of marketing campaigns on sales, the effect of temperature on crop yields, or the relationship between exercise and heart health, understanding the relationship between variables is essential for gaining insights and informing decision-making processes.
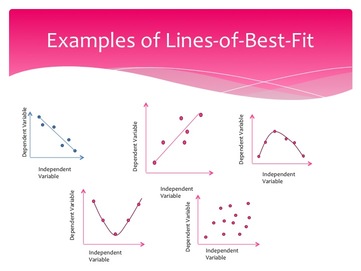
Understanding The Concept Of Relationship Between Variables
In statistics, the relationship between variables refers to the association or connection between two or more variables. Variables can be any measurable quantities or characteristics that can vary.
Variable | Description |
Independent Variable | A variable that is manipulated or controlled in an experiment to observe its effect on the dependent variable. |
Dependent Variable | A variable that is expected to change as a result of the independent variable. |
Confounding Variable | A variable that affects the relationship between the independent and dependent variable, leading to a false association. |
Understanding the relationship between variables is crucial in statistical analysis as it helps in making predictions, drawing conclusions, and understanding the impact of one variable on another. It involves exploring patterns, correlations, and causal relationships. Different statistical techniques and tools are used to analyze and quantify the relationship between variables, such as correlation coefficients, regression analysis, and hypothesis testing.
By carefully examining the relationship between variables, researchers and analysts can gain valuable insights, make informed decisions, and contribute to the advancement of various fields.
Types Of Relationships Between Variables
Positive relationships, negative relationships, no relationship (or zero relationship), curvilinear relationships, moderating relationships, and mediating relationships are the different types of relationships that can exist between variables.
Type of Relationship | Description |
---|---|
Positive Relationships | When there is a positive relationship between variables, an increase in one variable leads to an increase in the other variable. |
Negative Relationships | On the other hand, a negative relationship means that an increase in one variable results in a decrease in the other variable. |
No Relationship (or Zero Relationship) | When there is no relationship or zero relationship between variables, changes in one variable do not impact the other variable. |
Curvilinear Relationships | Curvilinear relationships occur when the relationship between variables is not linear but instead follows a curve or a curved line. |
Moderating Relationships | In moderating relationships, the relationship between two variables is influenced or modified by a third variable. |
Mediating Relationships | Mediating relationships exist when the relationship between two variables is influenced by a third variable that acts as a mediator or a go-between. |
Exploring The Strength And Direction Of Relationships
Relationships between variables can provide valuable insights and help us understand the dynamics and patterns within data. Measuring the strength of relationships allows us to determine the extent to which two variables are related. This can be done using correlation coefficients, such as the Pearson correlation coefficient, which ranges from -1 to +1. A value close to -1 or +1 indicates a strong relationship, while a value close to 0 suggests a weak relationship.
The direction of relationships refers to whether the variables move in the same direction (positive relationship) or in opposite directions (negative relationship). A positive relationship means that as one variable increases, the other variable also increases, whereas a negative relationship indicates that as one variable increases, the other variable decreases.
For example, a strong positive relationship could be observed between the amount of time spent studying and exam scores. On the other hand, a weak negative relationship may exist between the number of hours spent playing video games and academic performance. Having a clear understanding of the strength and direction of relationships between variables allows us to make informed decisions and predictions based on data.
Correlation Analysis
Correlation analysis examines the relationship between two or more variables to determine if there is a statistical association between them. It is used to measure the strength and direction of the relationship, and can provide valuable insights in various fields, such as finance, psychology, and social sciences.
Introduction To Correlation
Correlation is a statistical measure that quantifies the extent to which two variables are related. It ranges from -1 to +1, where -1 represents a perfect negative correlation, +1 represents a perfect positive correlation, and 0 represents no correlation. A positive correlation means that as one variable increases, the other variable also tends to increase, while a negative correlation indicates that as one variable increases, the other variable tends to decrease.
Types Of Correlation Coefficients
There are several types of correlation coefficients, with the most commonly used being Pearson’s correlation coefficient, Spearman’s rank correlation coefficient, and Kendall’s rank correlation coefficient. Pearson’s correlation coefficient is used when both variables are continuous and follow a normal distribution, while Spearman’s and Kendall’s correlation coefficients are used when the variables are ordinal or not normally distributed.
Interpreting Correlation Coefficients
Interpreting correlation coefficients involves considering both the magnitude and direction of the correlation. A coefficient close to +1 or -1 indicates a strong relationship, while a coefficient close to 0 suggests a weak or no relationship. The direction of the coefficient indicates the positive or negative nature of the relationship. However, it is important to note that correlation does not imply causation, and other factors may be influencing the relationship between the variables.
Regression Analysis
The relationship between variables is a fundamental concept in regression analysis. Understanding this relationship is crucial for making meaningful interpretations and predictions based on data. Regression analysis involves examining the relationship between a dependent variable and one or more independent variables.
Regression analysis is a statistical technique used to model and analyze the relationship between a dependent variable and one or more independent variables. It helps in understanding how changes in independent variables affect the dependent variable. This analysis is widely used for predictive modeling, forecasting, and understanding causality.
Simple linear regression focuses on studying the relationship between one independent variable and a dependent variable. It uses a linear equation to represent the relationship between these variables. The equation helps to estimate the value of the dependent variable based on the given independent variable.
Multiple regression extends the concept of simple linear regression by considering more than one independent variable. It allows us to understand how multiple variables collectively contribute to the variation in the dependent variable. This analysis helps in identifying the significant variables and their individual impacts on the outcome.
The interpretation of regression results involves analyzing the coefficients, p-values, and confidence intervals. These provide insights into the strength, direction, and significance of the relationship between variables. The interpretation allows researchers and practitioners to make informed decisions based on the regression model’s output.
Causal Relationships
Establishing causality in research studies is an essential aspect of understanding the relationship between variables. When investigating the causal relationships between variables, researchers strive to determine if changes in one variable directly influence changes in another. This process involves identifying potential confounding variables that may affect the outcome of the study.
Confounding variables can hinder causal inference by introducing extraneous factors that may influence the relationship between the variables of interest. To mitigate this issue, researchers employ various methods such as randomization, control groups, and statistical techniques to minimize the impact of confounding variables.
Key Points: |
---|
Establishing causality in research studies is crucial. |
Confounding variables can potentially affect causal inference. |
Randomization, control groups, and statistical techniques help mitigate the impact of confounding variables. |
Business And Marketing
The relationship between variables is crucial in business and marketing. Market research plays a significant role in understanding consumer behavior. It helps businesses identify patterns and trends that can influence their marketing campaigns and ultimately impact their Return on Investment (ROI). By conducting thorough market research, businesses can gather valuable insights into their target audience’s preferences, needs, and buying behavior. This information can then be used to create targeted and effective marketing campaigns that resonate with consumers, resulting in a higher ROI. Understanding the relationship between variables such as consumer demographics, psychographics, and purchase behavior can provide businesses with a competitive edge in the market. It allows them to develop tailored strategies that align with their target audience’s preferences and drive business growth.
Health And Medicine
In the field of health and medicine, understanding the relationship between variables is crucial. Clinical trials are conducted to assess the effectiveness of a treatment or intervention. These trials involve gathering data from a group of participants and comparing the outcomes between those who receive the treatment and those who do not. By analyzing the data, researchers can determine the treatment effectiveness and make informed decisions about patient care.
Epidemiology is another important area of study in health and medicine. It focuses on identifying factors that contribute to the development and spread of diseases. By studying patterns and risk factors, epidemiologists can better understand disease prevalence and disease risk factors. This information is valuable in developing strategies for prevention and control.
Overall, the relationship between variables in health and medicine is complex and multifaceted. Through clinical trials and epidemiological studies, researchers can gain insights into treatment effectiveness and disease risk factors, ultimately leading to improved patient care and public health outcomes.
Social Sciences And Education
When studying educational research and student achievement, it is important to understand the relationship between variables. Variables in educational research refer to the factors or characteristics that can vary and potentially impact student outcomes. These variables might include factors such as student motivation, teacher qualifications, parental involvement, and classroom resources.
Understanding the relationship between variables helps researchers identify patterns and make informed conclusions. For example, a study might aim to determine the relationship between teacher qualifications and student achievement. By analyzing data and examining variables such as teacher education level, years of experience, and student test scores, researchers can gain insights into how these factors might influence student success.
By systematically studying and analyzing the relationships between variables, educational researchers can uncover valuable insights that can inform educational practices and policies. This knowledge can contribute to improving student achievement and overall educational outcomes.
Does the Relationship of Command Concept Have Similarities to the Relationship Between Variables?
The understanding relationship of command is similar to the relationship between variables in that both involve a hierarchical structure. In the relationship of command, there is a clear authority, while in the relationship between variables, there is a dependent and independent variable dictating the connection.
Frequently Asked Questions For What Does Relationship Between Variables Mean
What Is An Example Of A Relationship Between Variables?
An example of a relationship between variables is that as the temperature increases, ice cream sales also increase.
How Do You Interpret The Relationship Between Two Variables?
To interpret the relationship between two variables, analyze how changes in one variable affect the other. Use statistical methods to measure the strength and direction of the relationship.
What Does The Relationship Between Variables Mean?
The relationship between variables refers to how changes in one variable affect another variable in a consistent and predictable way.
Conclusion
To summarize, understanding the relationship between variables is essential for analyzing data and making informed decisions. By recognizing the connections and patterns between different factors, we can gain valuable insights into their impact on one another. This understanding not only helps us identify causation or correlation but also aids in predicting future outcomes.
By leveraging this knowledge, we can optimize processes, develop effective strategies, and drive successful outcomes in various fields. So, never underestimate the power of understanding the relationship between variables.